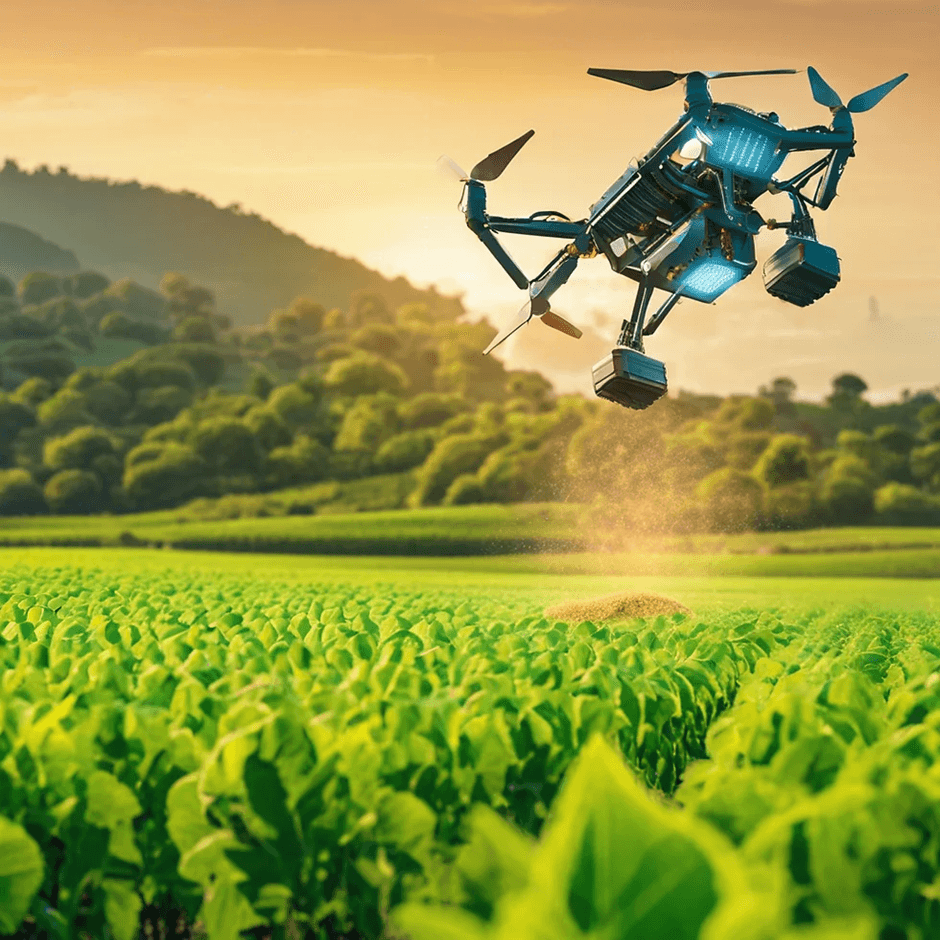
Leveraging Machine Learning to Improve Kenya’s Agricultural Yield in 2025
Introduction
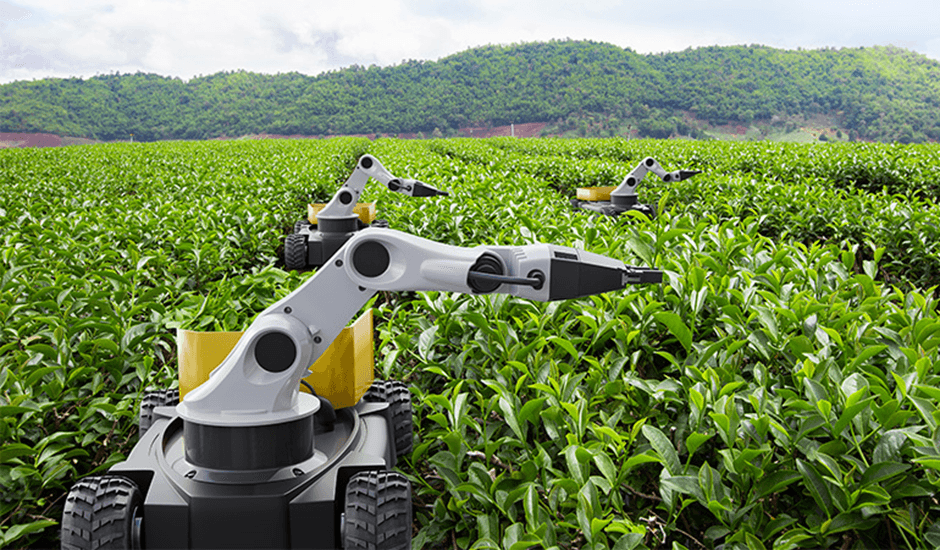
Agriculture is the backbone of Kenya’s economy, contributing approximately 25% of the country’s GDP and employing nearly 70% of the population. Despite its critical role, the sector faces significant challenges, including unpredictable weather patterns, soil degradation, pests, and inefficient farming practices. In 2025, machine learning (ML) is emerging as a transformative tool, helping farmers optimize agricultural yields, reduce waste, and make data-driven decisions.
In this blog, we’ll explore how machine learning is being applied to improve Kenya’s agricultural productivity, the benefits it offers, and the challenges and opportunities it presents for the future.
How Machine Learning is Revolutionizing Agriculture in Kenya
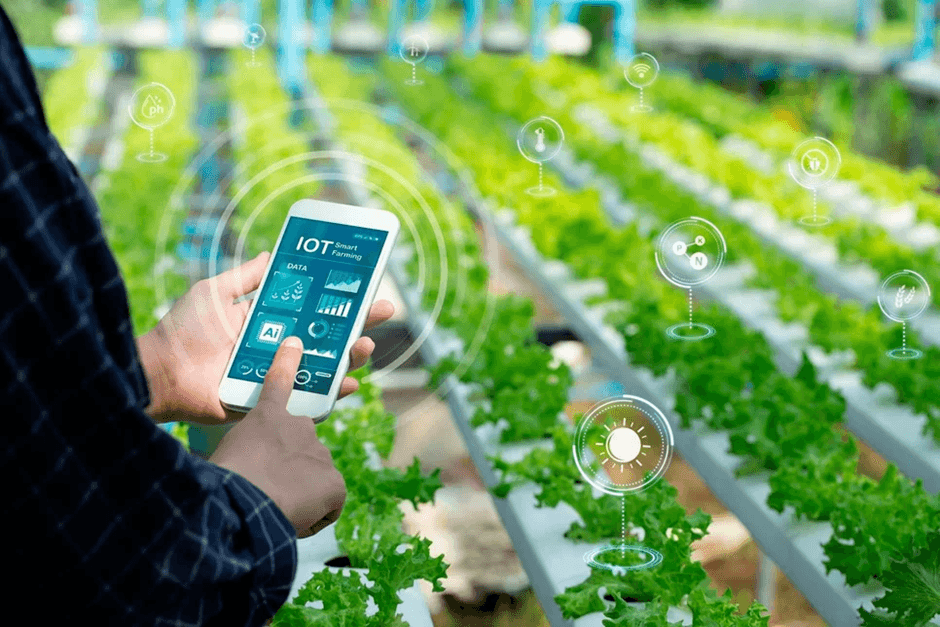
- Precision Agriculture
Machine learning algorithms analyze satellite imagery, drone data, and IoT sensor inputs to provide insights on soil health, crop conditions, and water requirements. Startups like Twiga Foods and CropIn are leveraging ML to offer farmers actionable insights, ensuring precise use of resources. - Pest and Disease Management
ML-powered apps can identify pests and diseases in crops by analyzing images uploaded by farmers. These systems, such as PlantVillage Nuru, recommend targeted interventions, reducing reliance on broad-spectrum pesticides and minimizing crop losses. - Weather Prediction
Advanced ML models are improving the accuracy of weather forecasts, helping farmers plan planting and harvesting schedules. Platforms like aWhere provide hyperlocal weather updates, allowing farmers to adapt to changing climatic conditions. - Yield Prediction
Machine learning tools analyze historical data and real-time inputs to predict crop yields, enabling better planning for storage, transportation, and market supply. This minimizes post-harvest losses and ensures farmers get fair prices for their produce. - Market Insights and Supply Chain Optimization
ML systems connect farmers with buyers through digital platforms, predicting market demand and prices. This helps farmers make informed decisions about when and where to sell their produce, reducing exploitation by middlemen.
Benefits of Machine Learning in Kenyan Agriculture
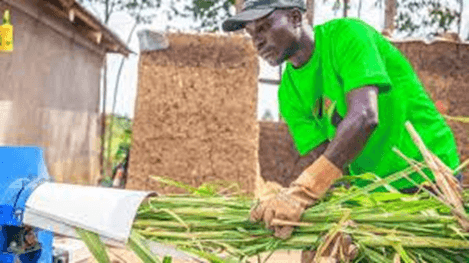
- Increased Productivity
By providing data-driven recommendations, ML enables farmers to maximize yields while minimizing input costs. - Sustainability
ML promotes sustainable farming by optimizing the use of water, fertilizers, and pesticides, reducing environmental impact. - Risk Mitigation
Predictive analytics help farmers anticipate risks such as droughts, floods, or pest outbreaks, allowing them to take preventive measures. - Empowering Smallholder Farmers
Affordable ML-powered tools make advanced technology accessible to smallholder farmers, who form the majority of Kenya’s agricultural workforce.
Challenges in Implementing Machine Learning in Agriculture
- Data Scarcity
Accurate ML models require large volumes of high-quality data, which can be difficult to obtain in regions with limited digital infrastructure. - Limited Digital Literacy
Many farmers lack the skills to use ML-based platforms, necessitating training and awareness programs. - Infrastructure Constraints
Poor internet connectivity and lack of access to smartphones or computers hinder the adoption of ML technologies in rural areas. - High Initial Costs
The cost of deploying ML tools, from sensors to software, can be prohibitive for small-scale farmers without external support or subsidies.
Opportunities for Growth in 2025
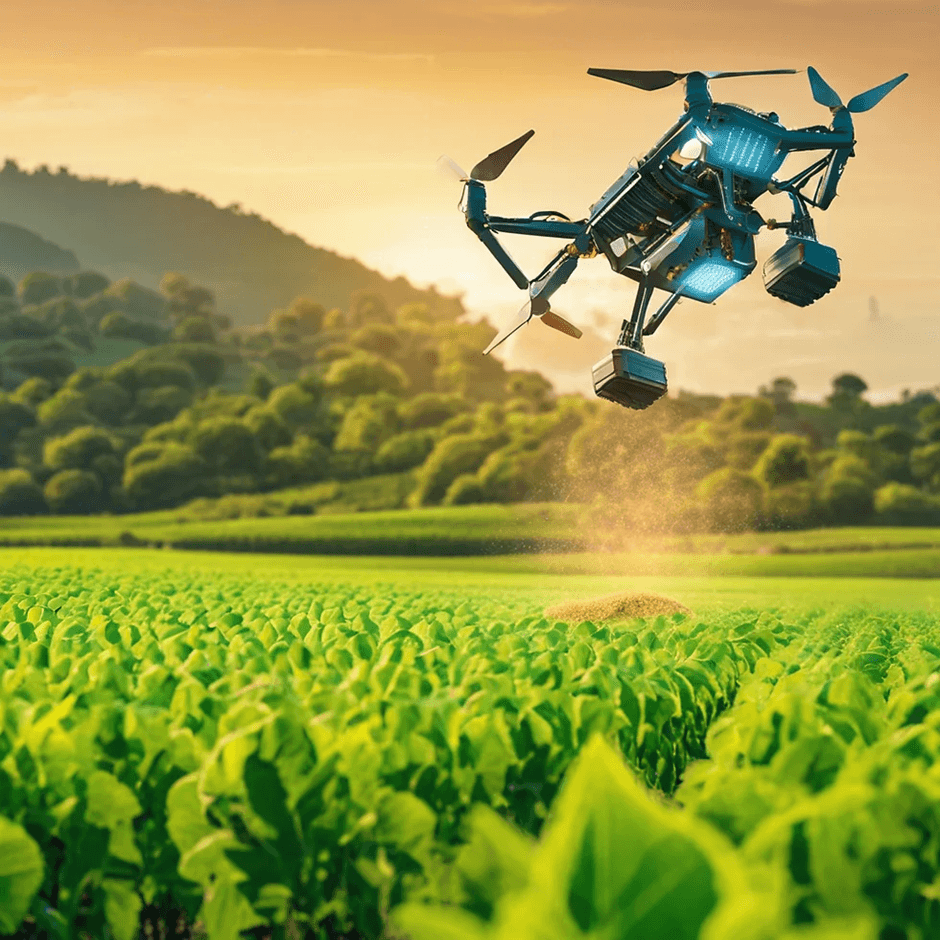
- Public-Private Partnerships
Collaborations between the government, tech companies, and agricultural organizations can drive investment in ML-powered tools and infrastructure. - Localized Solutions
Developing ML models tailored to Kenya’s unique agricultural landscape can improve their accuracy and relevance. - Integration with Mobile Platforms
As mobile penetration increases in Kenya, ML tools integrated into apps like M-Farm can reach a wider audience. - Export Potential
By boosting yields and ensuring quality, ML can help Kenyan farmers meet export standards, increasing their access to international markets.
Conclusion
Machine learning is poised to transform Kenya’s agricultural sector in 2025, offering innovative solutions to age-old challenges. By enabling precision farming, improving pest management, and providing actionable market insights, ML is empowering Kenyan farmers to achieve higher yields, reduce waste, and increase their income.
However, to fully realize its potential, stakeholders must address barriers such as data access, digital literacy, and infrastructure. With the right investments and policies, machine learning can drive Kenya towards a more sustainable and prosperous agricultural future.
References